Lessons Learned About Data Analytics In An Intro Session At General Assembly
Data Analytics and Data Science are two hotly discussed topics on the inter-webs these days. I’ve been interested in them as professional…
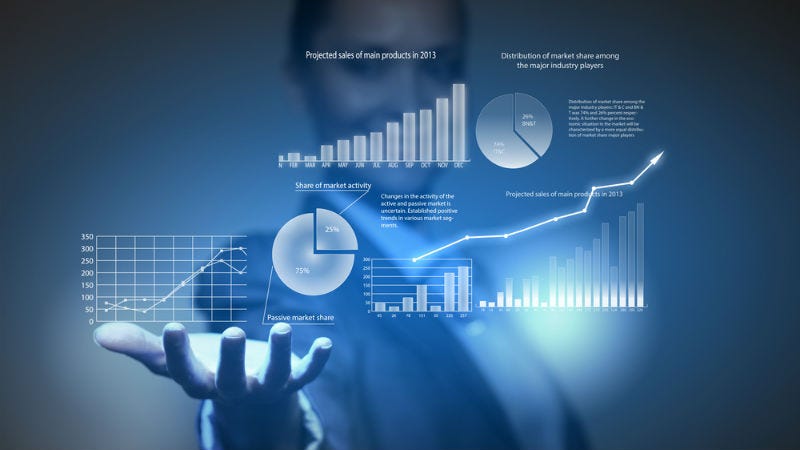
Data Analytics and Data Science are two hotly discussed topics on the inter-webs these days. I’ve been interested in them as professional fields since I discovered their career demand and profitability and my aptitude in particular parts of the fields. I’ve obsessively read about how to get started and how to land a job in the field. But, I never made it a priority to do so with unrelenting persistence. And, even now I still haven’t. I’m a full stack software engineer, and for the moment, I’m working on doing that really well.
I’ve started and stopped enough online courses to discuss the basic topics intelligently, but I have no real experience with the subjects. I’ve exposed myself to data science tools, workflows, and have subscribed to a lot of related content streams. But, in order for me to progress with this skill-set, I’ll need to move beyond shallow engagements.
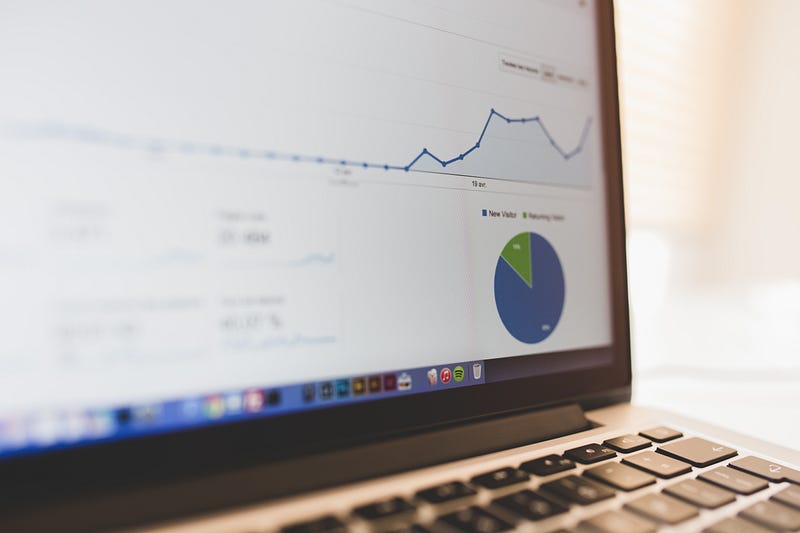
Last summer I met Otis, the Director of Analytics at Clover Health, and he shared some resources with me after I expressed my interest in data science. I went through much of the resources over the summer, but haven’t worked with the topics since. I prioritized my school work and other life responsibilities over my interest in data science. A few days ago I saw a General Assembly ad on Facebook with an invitation to a free 2 ½ hour Intro to Data Analytics.
The spots were already filled, but I decided to show up at the venue early in the hopes that either someone had cancelled or I could just crouch on the floor with my laptop and take in some knowledge. Well, that worked! I attended the session last Wednesday, but I soon realized I was beyond this point of introduction. I stayed the full length of the session hoping to learn something, meet some people, or at the very least discover anything that might provide me with some value. Fortunately, I did get something of value from attending the session.
We began by establishing the reasons why data analytics is relevant and communicating our motivations for wanting to learn about data analytics. It turns out that knowledge of data can be utilized in every industry and that data must be interpreted to become information so that we can gain value from it. But, I already knew that, so let’s keep going. We then defined some key terms. Among them were sample data, population data, small data, big data, data science, and data analytics. We also spoke about some of the tools that are often used to work with data, tools including Python, R, Microsoft Excel, Google Sheets, and SQL.
We the looked at a familiar data analysis workflow. Define the problem or goal. Create or find the data. Prepare and clean the data. Analyze the data. And, visualize the data and make recommendations which include stating the results and assumptions made, interpreting the results and drawing conclusions, and considering shortcoming of the data and outline a plan for future analysis.
Still, much of this is old news to me, so you might be wondering what value did I get from attending this session? Stick with me a while longer. The value came from actually doing the analyses. We worked together to use the aforementioned workflow to analyze three data sets. That, and there was a hire me board outside the session. But, let’s get back to talking about data analysis.
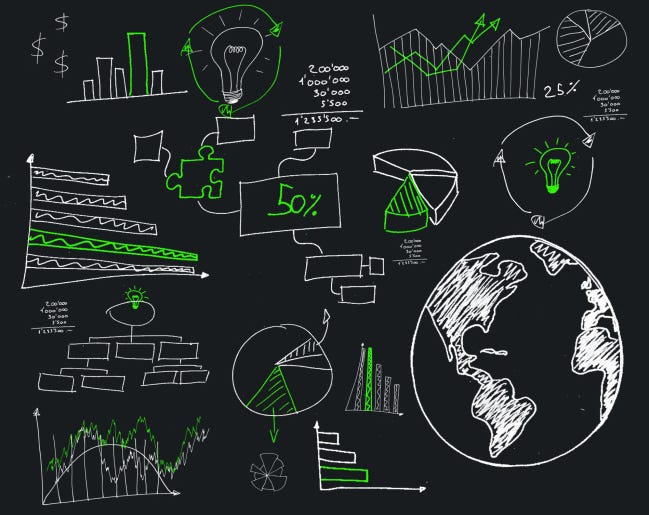
Our instructor gathered some data from each of the attendees in Google Sheets and walked us through some basic formulas including MIN, MAX, AVERAGE, MEDIAN, COUNT, COUNTA, COUNTIF, FILTER, SUM, SUMIF, and UNIQUE. Then we worked together to analyze a movie data set, with the aim of making a smart bet on which type of movie would gross the highest revenue in 2017.
Afterwards, we were left on our own to analyze a data set containing the net worth of past presidents to find out if we are electing wealthier presidents with each election cycle. Doing these exercises really showed me how important it is to be transparent about the assumptions made and the steps taken in the data analysis process. Everyone pretty much had different takes on which methods to employ when cleaning the data and choosing their next logical steps in working with the data. Additionally, it became apparent that no data set will be perfect, and consequently, no data analysis will be perfect either.
Furthermore, a data set may need multiple other data sets to complement its data in order for the analyst to conduct a thorough analysis. These are important things to keep in mind in a time when we are continuously bombarded with statistics and all kinds of metrics and reports. Your numbers look fancy, but if you really want to impress me show me how you got them.
So, there. I had some fun analyzing data and learning about some of the ways that an analysis might be incomplete or in need of further revision. The main value I got from attending the session was using the previously mentioned workflow to analyze the data. And, remember that hire me board? I put my name down. If you’re looking for a curious, ever growing, and fun to work with engineer, check me out.
If you liked this, click the💚 below so other people will see this here on Medium.